Driving IT Accountability and AI Efficiency
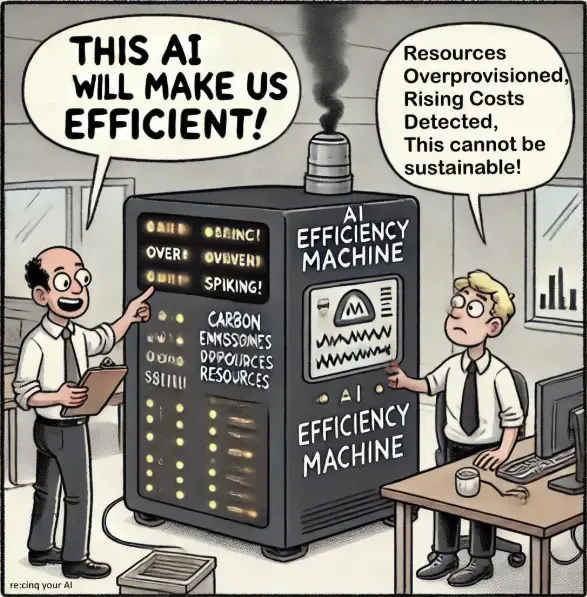
Enterprises today face heightened scrutiny over their operational expenses, energy consumption, and carbon footprints. Research from multiple industry sources including the FinOps Foundation and Gartner reveals that software licensing, underutilized resources, and lack of carbon transparency often combine to drive up costs and environmental impact. An IT accountability strategy that integrates cost optimization, resource efficiency, and sustainability can help organizations align financial, operational, and environmental objectives. This overview examines core challenges and best practices to address them.
Software Licensing and Usage Optimization
Common Challenges
Enterprises frequently grapple with unwieldy licensing terms, decentralized purchasing, and limited visibility into actual usage. These issues lead to:
- Unexpected True-Up Costs: When actual software usage surpasses licensed amounts, unbudgeted expenses may arise during renewal cycles.
- Over-Provisioned Licenses: Inactive or underutilized seats inflate costs without contributing to productivity.
- Compliance Risk: Insufficient tracking mechanisms can trigger penalties or legal concerns if usage consistently exceeds license terms.
Best Practices
- Centralized License Management: Implement unified tools for tracking usage, automating renewal reminders, and consolidating licensing data.
- Cross-Functional Collaboration: Involve finance, procurement, and IT operations in periodic audits to assess whether licensed software aligns with current business needs.
- Usage Analytics and Automation: Use monitoring solutions that generate real-time alerts if usage nears the licensed threshold, allowing teams to either reduce consumption or negotiate expanded terms.
A 2024 FinOps Foundation report highlighted that organizations with proactive, automated license tracking can save up to 25% on software expenses annually, simply by eliminating redundant or idle licenses (FinOps.org ).
Tracking and Accountability
Common Challenges
Although 65% of Global 2000 companies have pledged carbon neutrality targets, fewer than half effectively measure IT-related emissions, according to a recent CDP (Carbon Disclosure Project) survey (CDP.net ). Lack of transparent data from software vendors and infrastructure providers complicates efforts to hold third parties accountable.
Best Practices
- Emissions Frameworks: Develop a standardized methodology aligned with frameworks like the Greenhouse Gas Protocol to calculate carbon output across servers, data centers, and cloud resources.
- Vendor-Linked Reporting: Generate detailed scorecards to pinpoint each software vendor’s contribution to the organization’s overall emissions footprint.
- Real-Time Emissions Monitoring for Anomalies: Carbon emissions tracking provides an immediate signal of inefficiencies, often before cost-based metrics detect them. Spikes in emissions can indicate overprovisioned workloads, misconfigured autoscaling, or excessive energy consumption.
- Negotiation Leverage: Use carbon metrics to demand transparency and reduce emissions from partners, potentially securing better service-level agreements (SLAs) or green energy commitments.
Gartner predicts that sustainability-linked vendor contracts will become a common practice, driving both cost savings and brand reputation gains. Accurately tracking carbon emissions is a catalyst for informed negotiations and meaningful reductions in environmental impact.
Resource Efficiency and Cost Optimization
Common Challenges
A 2024 report by Virtana found that despite high confidence levels in understanding and managing their hybrid cloud environments, 87% of respondents are chronically overprovisioned, underprovisioned, or both (Virtana.com ).
Best Practices
- Workload Analysis: Examine usage patterns to right-size container instances, storage allocations, and virtual machines.
- AI-Driven Autoscaling: Implement machine learning algorithms that automatically adjust resource allocation based on real-time metrics, application load, and performance thresholds.
- Carbon Emissions as a Real-Time Efficiency Anomaly Detector: Carbon tracking tools can expose inefficiencies faster than cost-based models, revealing areas of waste that traditional monitoring may miss.
- Holistic Cost-Vs.-Performance Reviews: Regularly evaluate whether workloads can be run in lower-cost environments (e.g., spot instances, on-premises) without compromising service quality.
AI Workloads and the Critical Need for Baseline Metrics
Why This Must Happen Before AI Adoption
- AI Workloads Are Resource-Intensive: Machine learning models and large-scale AI deployments require vast computational power, leading to unpredictable spikes in cost and energy use.
- Lack of Pre-AI Baseline Metrics: Without clearly defined efficiency baselines before AI deployment, organizations may fail to track whether AI optimizations are actually improving operations or simply shifting inefficiencies elsewhere.
- AI’s Black Box Nature: Many AI-driven decision-making processes are opaque, making it difficult to pinpoint inefficiencies without independent baseline data.
- AI Can Mask Inefficiencies: AI applications may generate dynamic workloads, making it harder to detect cost overruns, waste, and emissions increases unless pre-deployment metrics exist.
Best Practices for AI Readiness
- Establish Pre-AI Efficiency Benchmarks: Track key resource utilization, cost, and carbon footprint metrics before deploying AI models to create clear reference points for future optimizations.
- Use Carbon Metrics to Detect AI Model Drift: AI models may gradually consume more resources as they evolve. Monitoring real-time emissions spikes can reveal model inefficiencies.
- Optimize AI Workloads for Sustainability: AI autoscaling models should incorporate carbon efficiency alongside traditional performance and cost metrics to prevent unnecessary energy use.
- Ensure Vendor Transparency: AI workloads often rely on external cloud providers, making vendor emissions tracking crucial for accountability and efficiency.
Monitoring and Validation Framework
Common Challenges
Some enterprises adopt new optimization strategies or carbon-tracking measures but fail to set baselines or verify the real-world effects of their changes. Without quantifiable before-and-after insights, potential gains in cost reduction or emissions control remain unclear.
Best Practices
- Initial Benchmarking: Collect metrics for software usage, carbon emissions, and resource allocation prior to any optimization or vendor negotiation.
- Post-Implementation Verification: Validate improvements after executing changes whether it’s license consolidation, resource right-sizing, or adopting carbon reduction targets.
- Ongoing Governance: Incorporate periodic reviews (monthly or quarterly) to ensure that short-term gains persist as application demands and vendor relationships evolve.
DevOps Research & Assessment (DORA) notes that continuous measurement of both performance and efficiency indicators fosters a culture of iterative improvement, ultimately boosting operational reliability.
Aligning Cost, Carbon, and Operational Goals
The evolving business landscape demands more than isolated cost-cutting measures; it calls for integrating financial accountability with sustainability imperatives. An IT accountability and efficiency approach holistically addresses these objectives by monitoring software usage, enforcing resource optimization, and holding both internal operations and external vendors accountable for carbon outputs. By treating carbon emissions as a real-time anomaly detector, organizations can proactively identify inefficiencies, reduce costs, and optimize sustainability initiatives in tandem.
By adopting these best practices, enterprises set the stage for enduring operational success and environmental stewardship, a critical balance in the modern digital economy.
AI-Ops: More Than Just Metrics
Is AI strategy just about tracking metrics, or is it designed for long-term efficiency and sustainability?
It’s not just about what gets measured, but what gets implemented and how. AI-Ops must be built with efficiency, sustainability, and vendor accountability embedded from the start. Beyond dashboards and reporting, architecture dictates whether AI remains an asset or becomes an uncontrolled cost center.
re:cinq architects AI-Ops for the real world where cost, carbon, and compute efficiency align with business strategy. By designing AI systems with sustainability and accountability at the core, re:cinq ensures AI investments deliver measurable value without hidden inefficiencies draining resources.
AI is the future. re:cinq makes it work.