The Rise of Agentic AI: A Deep Dive
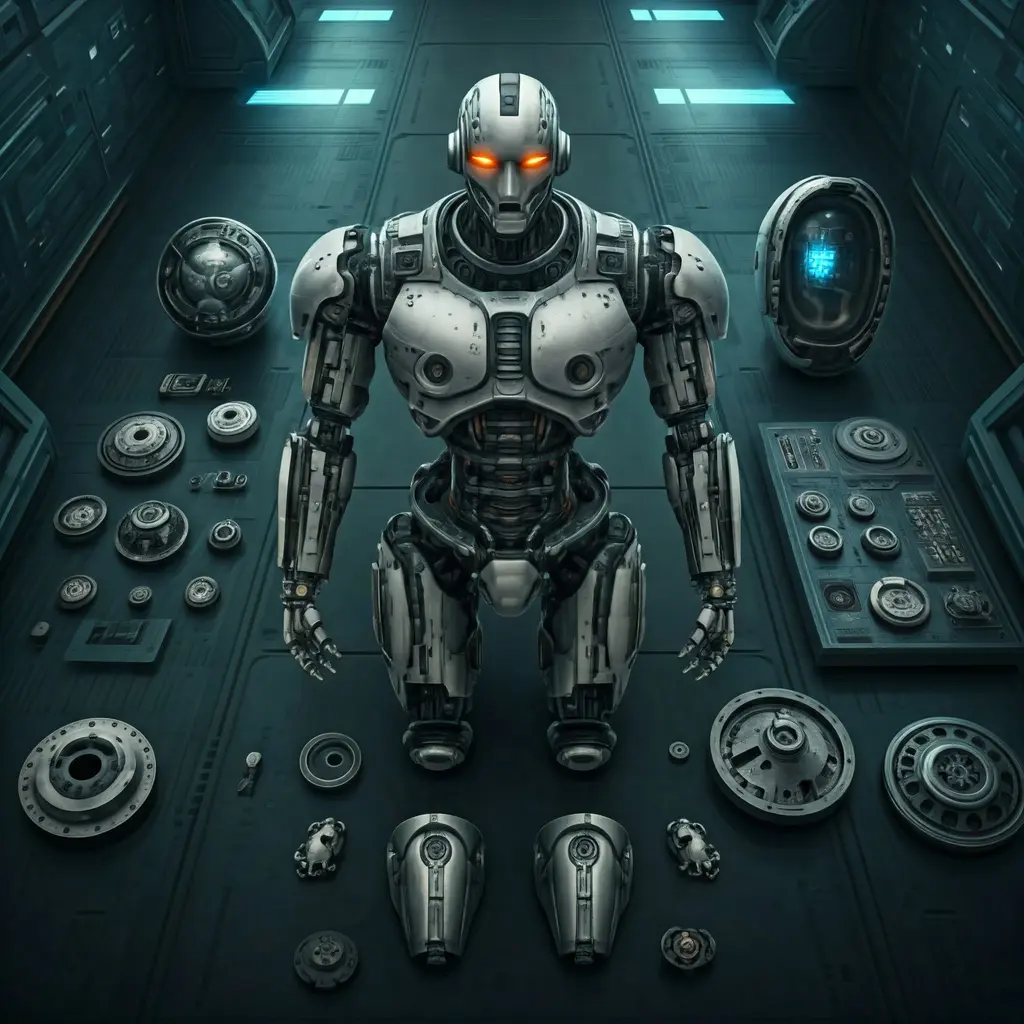
Artificial Intelligence continues to evolve at a rapid pace, and with it comes the rise of Agentic AI, another shifts in what machines can achieve. Unlike traditional systems that require predefined rules and static programming, Agentic AI brings autonomy, adaptability, and independent decision-making.
In this post, we’ll explore the underpinnings of Agentic AI and its implications across industries poised to be reshaped by this transformative technology.
Agentic AI: Moving Beyond Rules
For years, AI systems have relied on fixed algorithms, which are great for repetitive tasks but fail when faced with unpredictable situations. Agentic AI redefines this by introducing systems that don’t just follow rules, they perceive, plan, adapt, and learn. This shift is made possible by several key technical pillars:
Perception: Seeing the World Through Sensors
Agentic systems often start with perception modules that process inputs from their environment. These modules leverage techniques like Convolutional Neural Networks (CNNs) for visual data or Recurrent Neural Networks (RNNs) for sequences. They can handle a variety of sensory inputs, from LiDAR and cameras to real-time text streams, creating a detailed picture of their surroundings.
Knowledge Representation: Understanding and Reasoning
Knowledge graphs, symbolic logic, and other sophisticated frameworks allow Agentic systems to store, organize, and reason about information. This isn’t just about data retrieval, it’s about understanding context, drawing conclusions, and making informed decisions.
Planning and Decision-Making: Charting a Path
The ability to plan is what makes Agentic AI autonomous. Techniques like Monte Carlo Tree Search (MCTS), reinforcement learning (RL), and evolutionary algorithms enable agents to evaluate possible actions, choose the best path forward, and adapt to changes along the way.
Learning: Adapting Over Time
Unlike traditional systems, which are static once deployed, Agentic AI improves with experience. By using reinforcement learning, these systems refine their strategies based on real-world feedback, adapting to changes in their environment and improving over time.
Multi-Agent Systems (MAS): Collaboration in Action
In complex scenarios, multiple agents often need to work together. Multi-agent systems tackle this challenge by addressing coordination, communication, and cooperation using approaches like game theory and distributed consensus algorithms.
Agentic AI in Action: Transforming Industries
Agentic AI isn’t just theoretical; it’s already reshaping industries. Let’s look at a few key examples:
Finance: Smarter Trading and Fraud Detection
In finance, Agentic systems can process vast datasets, from market data to news sentiment, in real-time. They employ reinforcement learning to optimize trading strategies and adapt to volatile conditions. Similarly, deep learning models enable these agents to detect and even predict fraud, making them indispensable in financial markets.
(Source: scet.berkeley.edu
)
Manufacturing: Intelligent Factories
Smart factories leverage networks of agentic machines. Each machine monitors its health, predicts maintenance needs, and coordinates with others to optimize production. The result? Increased efficiency, reduced downtime, and greater adaptability in manufacturing processes.
(Source: scet.berkeley.edu
)
Transportation: Autonomous Mobility
Self-driving cars and drones embody Agentic AI at its best. With perception systems powered by CNNs and advanced path-planning algorithms, these agents make real-time decisions to navigate traffic, avoid obstacles, and ensure passenger safety. Reinforcement learning further refines their driving behavior over time.
(Source: scet.berkeley.edu
)
Challenges and Ethical Considerations
As exciting as Agentic AI is, it also presents challenges:
Explainability and Transparency
The decision-making processes of these systems can be opaque, raising questions about accountability. Research into explainable AI (XAI) is essential to build trust and ensure responsible use.
Safety and Robustness
For safety-critical applications like healthcare or transportation, ensuring predictable and reliable behavior is paramount. This requires rigorous testing, formal verification, and robust design principles.
Ethics and Society
The autonomy of Agentic AI brings ethical dilemmas. Who’s responsible for an agent’s decisions? How do we ensure systems remain unbiased? And what happens to jobs when machines can do more than ever before? These are questions we must grapple with as a society.
A Thoughtful Conclusion
Agentic AI isn’t just a technological leap, it’s a rethinking of what machines can do. By combining perception, reasoning, planning, and learning, these systems are poised to revolutionize industries, from finance to manufacturing and beyond.
But with great power comes great responsibility. As we push the boundaries of autonomy, it’s crucial to address the ethical, safety, and transparency challenges that come with it. If we do, Agentic AI can become a cornerstone of a future where humans and intelligent agents collaborate to solve the world’s toughest problems.